Improving the effectiveness of Artificial Intelligence by using the A/B testing method: the case of Kliper.cl part 2
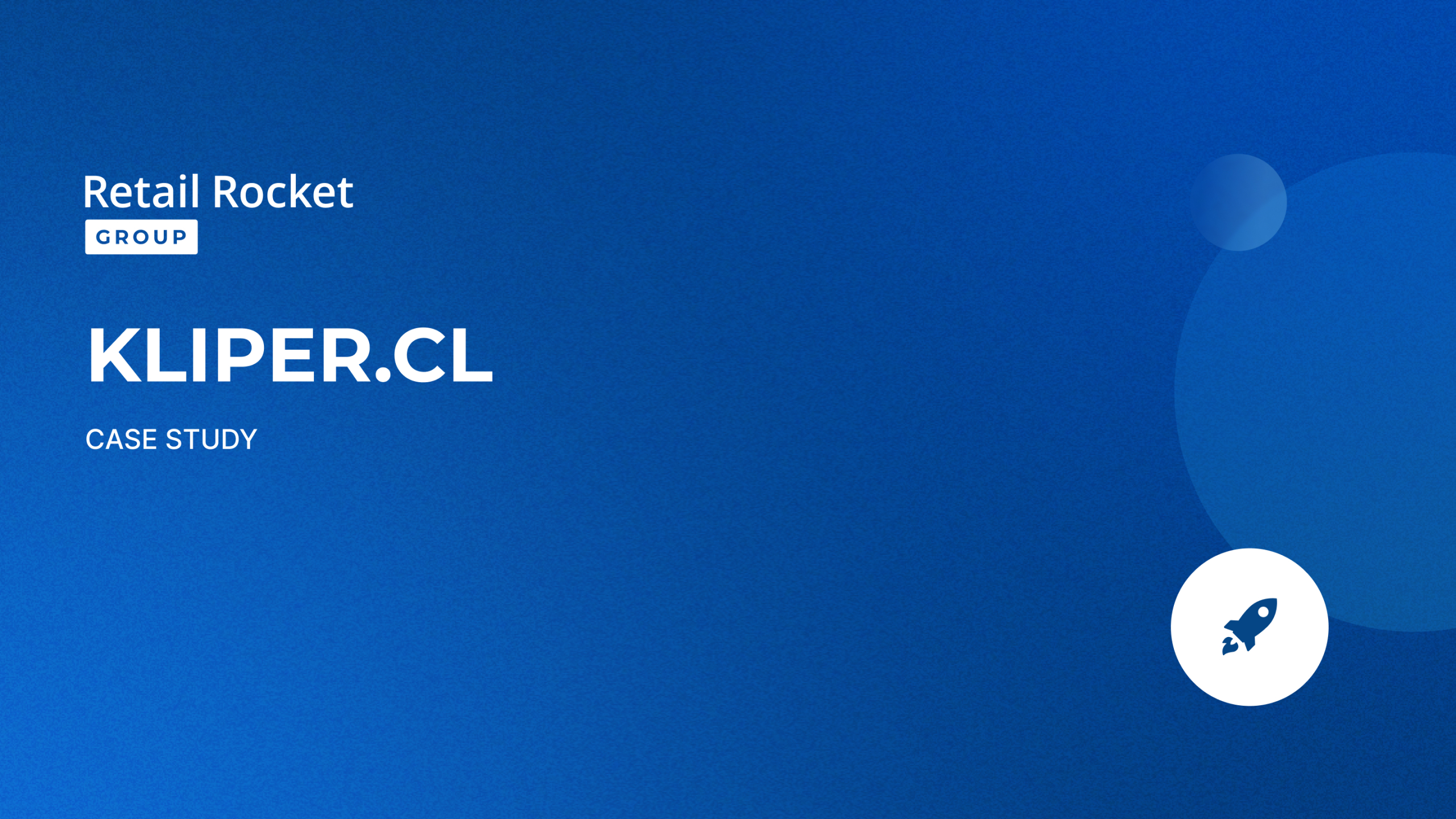
In the previous case study about Kliper, we saw how the multi-brand online store managed to increase the orders by more than 20% thanks to the AI implementation on the website and email marketing. In the present case, we will focus on how the effectiveness of this technology improved further thanks to the generation of hypotheses through A/B testing methodology.
About Kliper
Kliper is an exclusive online store with a vast range of fashion and outdoor products for the whole family. It is part of Komax, a retail company that represents major brands of clothing, accessories, and footwear, both in Chile and Peru. In its wide assortment, you will find firms such as The North Face, Kipling, DC Shoes, Brooks Brothers, UGG, Gap, Marmot, Mammut, among others.
The online store initial goals
By using the Retail Rocket’s technology, Kliper achieved to meet the objectives initially set:
- To create a unique shopping experience that facilitates the purchase decision in an online store with a wide assortment of products and brands.
- To increase customer retention and loyalty.
- To boost KPIs: conversion, average order value, and revenue.
With a personal approach both on the website and in the email channel, the online store obtained the following results:
- To increase the orders by 20.8%.
- To increase the online store’s revenue by 14.28%.
- An Average order value increase by 6.9% compared to the average on the site.
- The conversion is 3.5 times higher on the website and 8.8 times on the email channel than the online store average.
Let’s see how Retail Rocket has managed to increase further the metrics already obtained on the site. How? By performing iterative improvements with A/B tests to optimize the effectiveness of Artificial Intelligence on the product page.
Retail Rocket Solution
The Retail Rocket solutions based on Big Data technology are always personalized to meet every client’s specific needs. To work day a day on improving the business results is one of our main goals, and to reach it, the Growth Hacking team plays a key role.
To improve the online store’s metrics day a day, we focused the strategy on increasing the effectiveness of recommendations on the product page for both mobile and desktop versions. At this Customer Journey’s stage, the recommendation blocks play an essential function in helping visitors make a buying decision, increasing conversion and average order value.
A/B Test results
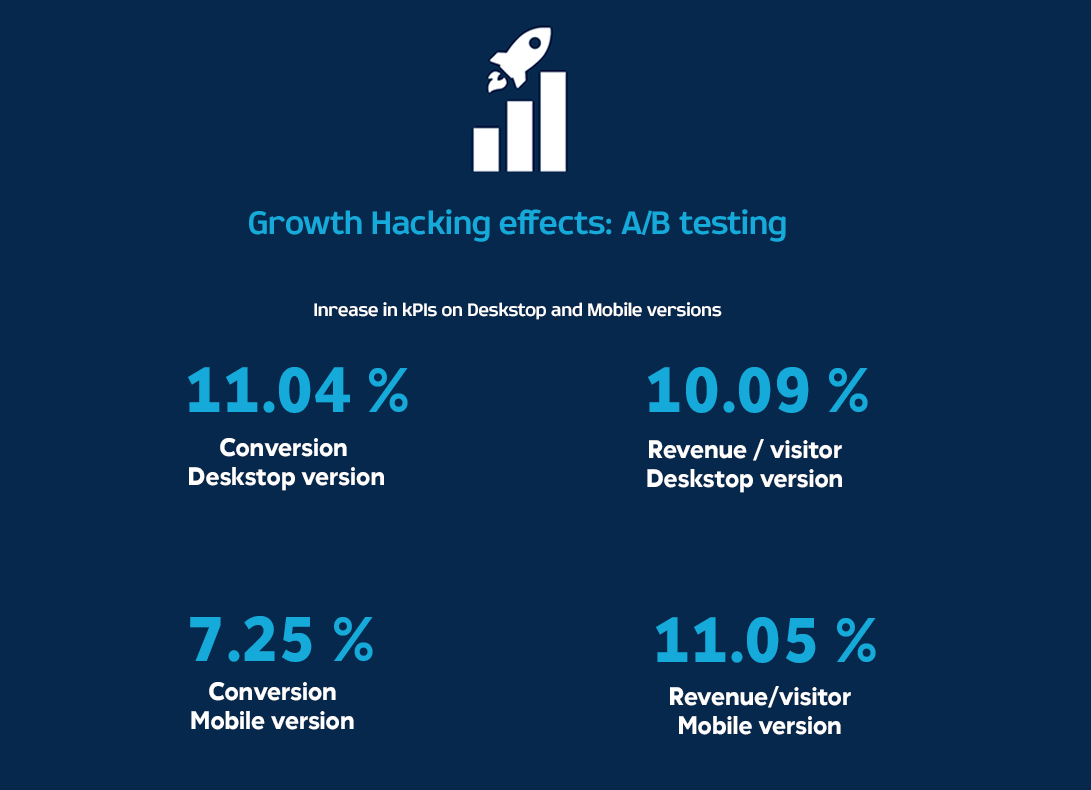
To improve the effectiveness of the personal recommendations on the product card page
Even what already works can be improved, and Retail Rocket, aware of this, never ceases to seek recurrent improvements to optimize the full potential of Artificial Intelligence in online stores using its technology.
The mechanics of A/B testing, through trial and error, is the perfect way to make data-driven decisions and increase the KPIs of your business.
The Growth Hacking specialists carried out these tests for Kliper online shop, analyzed during 30 days the performance of various configurations of recommendations on the product card page for the mobile and desktop versions, and chose the most effective one.
Hypothesis: changing the order of the recommendation blocks
The order of the recommendation blocks might affect the decision-making process. Thus showing first alternative products might make the process easier since it would be logical that a user decides by comparing products with similar characteristics before adding additional items to the cart.
While in mathematics it is true that the order of the summands does not alter the total, we will see below how the tests show that the recommendation blocks’ location does vary the result.
Desktop version
Here is below the data cleansing we used for the analysis of all A/B tests for avoiding errors:
● Visitors with several segment IDs are removed
● Visitors whose user agent shows that they are bots are removed
● Visitors who made orders via mobile phones and tablets are removed
● 3% of visitors with the highest number of orders are removed
● 3% of the orders with the highest revenue are removed
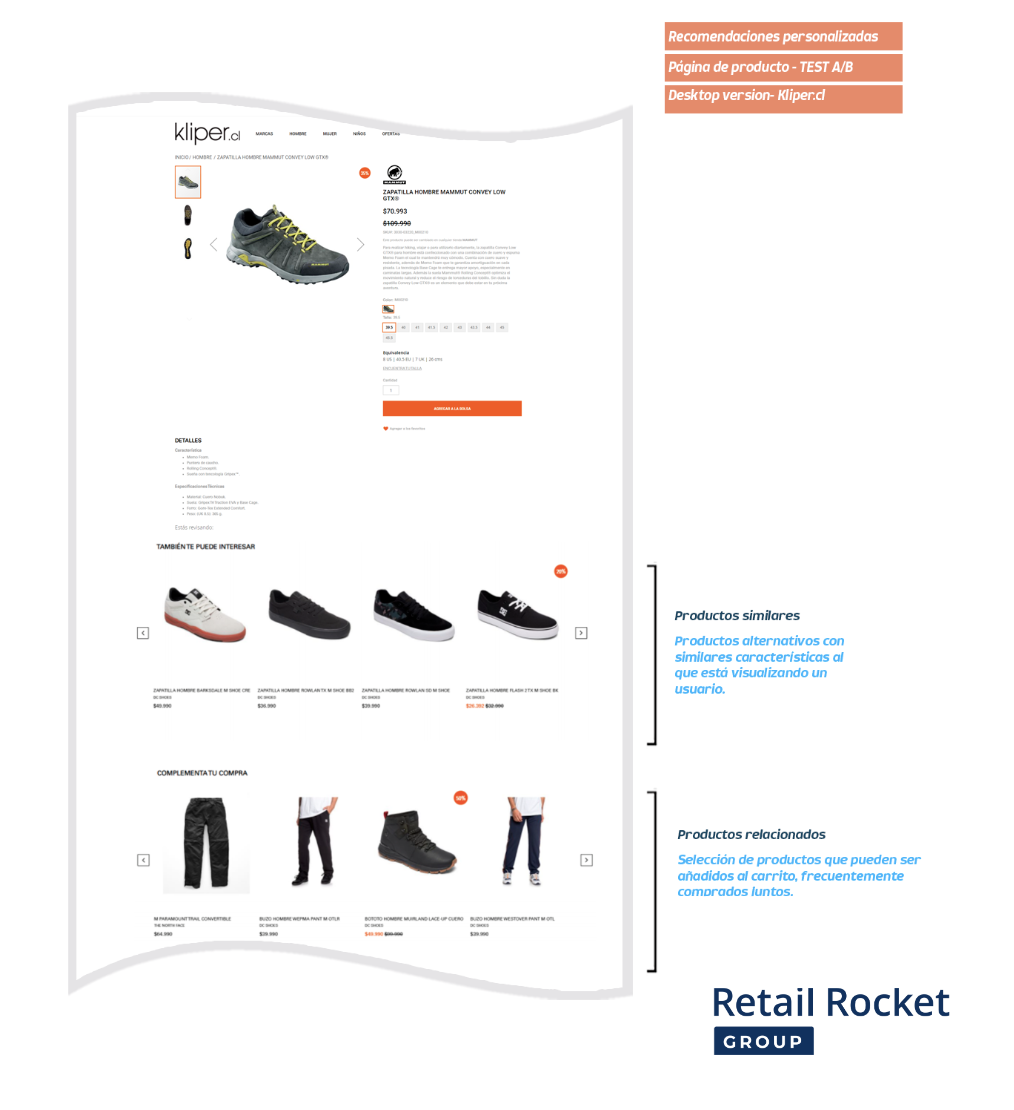
The visitors of the Kliper’s product page in the desktop version were randomly split into 3 segments in real-time:
The first segment, “Alternative + Related,” was shown a block with similar products followed by a selection of frequently purchased items together with the product a user is viewing. Both blocks contain personalized recommendations based on the user’s real-time interests and behavior.
The second segment, “Related + Alternative,” was shown first a block with products that are frequently purchased together with the item in the product card, followed by a selection of alternative options. Both blocks include personal recommendations based on the user’s interests and behavior in real-time.
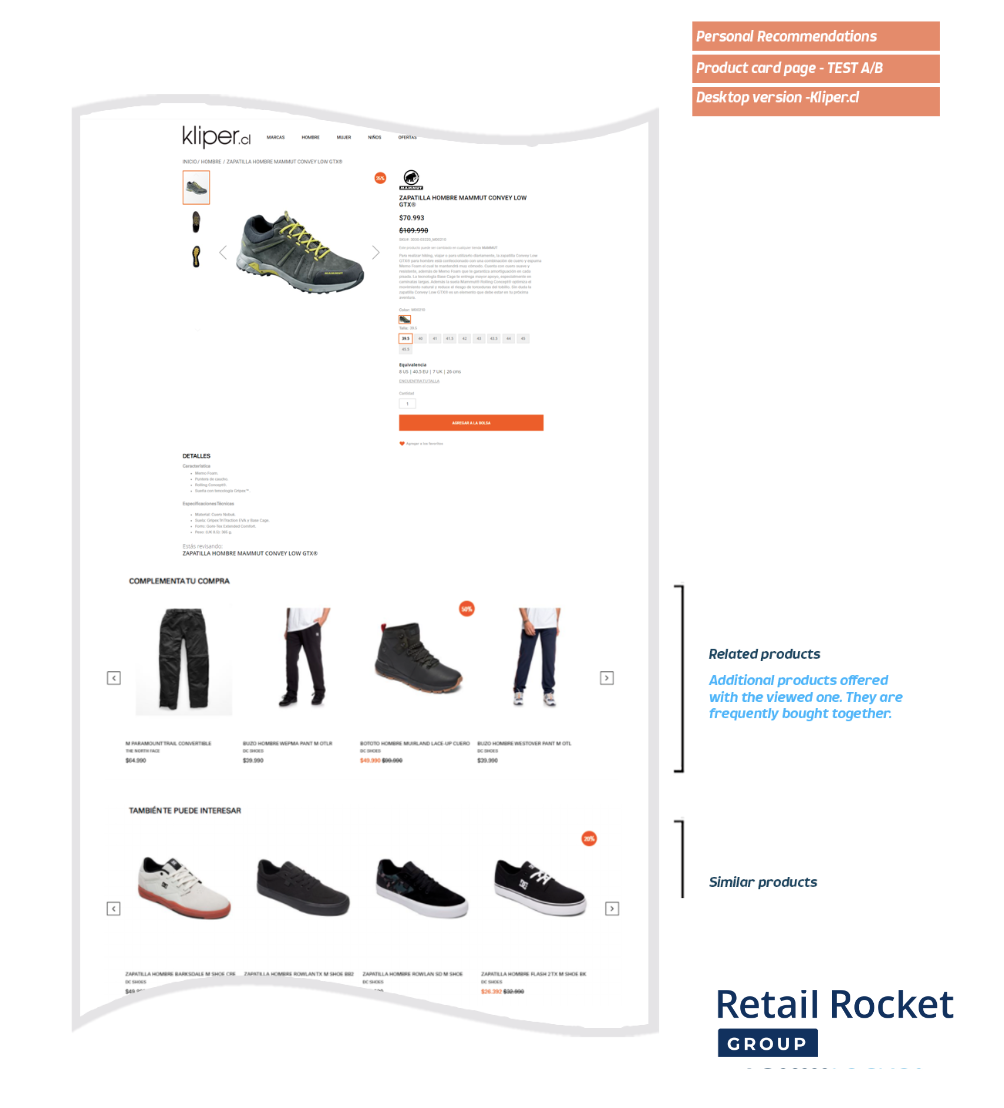
The third segment, the control group, was shown no recommendations
Results for the desktop version
Segment | Conversion(CR) | Average order value (AOV) | Revenue per visitor (RPV) | Revenue |
Alternative + Related | +11.04% | -0.86% | +10.09% | +8.85% |
Related + alternative | -3.63% | +4.38% | +0.52% | +3.64% |
Without recommendations | – | – | – |
The first segment, “Alternative + Related,” obtained the best results with an 11.04% increase in conversion and 10.09% growth in revenue per visitor, compared to the control group, which was not shown recommendations.
In both segments, displaying personal product blocks resulted in an increase in revenue compared to the absence of recommendations. That proves the effectiveness of AI technology in increasing sales.
Mobile version
We cleaned data used for the analysis of all A/B tests for avoiding errors:
● Visitors with several segment ids are removed
● Visitors whose user agent shows that they are bots are removed
● Visitors who made orders via desktops are removed
● 1% of visitors with the highest number of orders are removed
● 3% of the orders with the highest revenue are removed
The visitors of the Kliper’s product card page on the mobile device were randomly divided into 3 segments in real-time:
The first segment, (A) “Alternative + Related”, was shown a similar product block and secondly a block with frequently purchased items with the one a user is looking at. All products displayed are personal recommendations based on the user’s interest and behavior in real-time.
The second segment (B), “Related + Alternative,” was shown a selection of items commonly bought together with the product the visitor is viewing. And following a second block with similar items. Both recommendation blocks contain personal products based on the user’s interests and behavior in real-time.
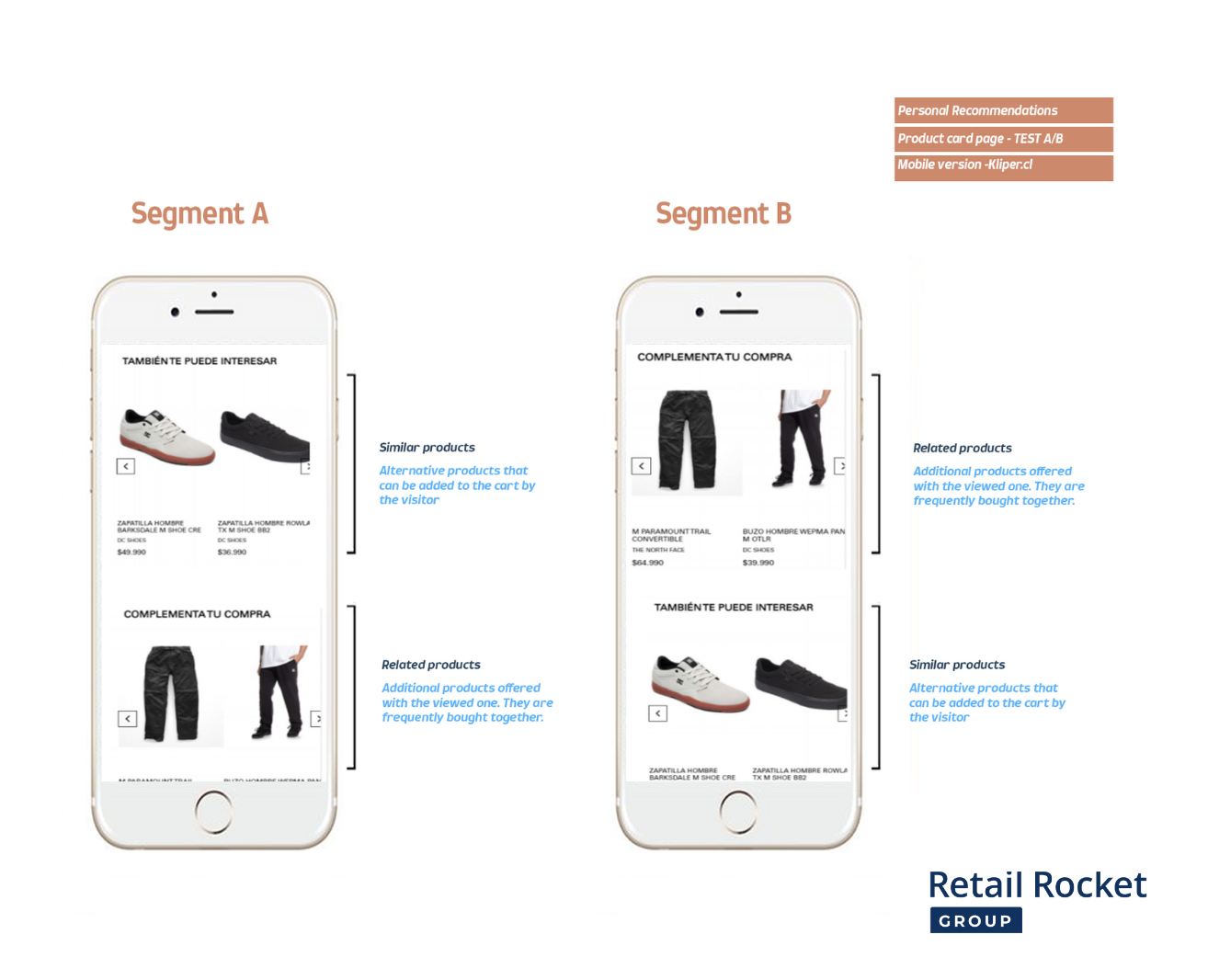
The third segment, the control group, was shown no recommendations.
Results for the mobile version
Segment | Conversion(CR) | Average Order Value (AOV) | Revenue per visitor (RPV) | Revenue |
Alternative + Related | +7.25% | +3.54% | +11.05% | +11.05% |
Related + Alternative | +3.02% | -0.22% | +2.73% | +4.67% |
Without recommendations | – | – | – |
The first segment, “alternative + related,” achieved the best results with a 7.25% growth in conversion and 11.05% increase in revenue per visitor, compared to the control group that was not shown recommendations.
The results obtained for both segments show the effectiveness of Retail Rocket’s personalization technology once again compared to the absence of recommendations.
Conclusions
The fact that a business already achieves excellent results does not imply that it should be limited to continuing to do the same thing over and over again. The Retail Rocket platform offers many solutions, and A/B testing is an example of how it is possible to improve what already works by maximizing the potential of AI personalization technology.
Changing the order of placement of the recommendation blocks on the product page of the Kliper online store resulted in:
- An 11.04% increase in conversion and 10.09% growth in revenue per visitor in the desktop version.
- 7.25% growth in conversion and 11.05% increase in revenue per visitor in the mobile version.
For both versions, it is more effective to display first an alternative product block, followed by a second block with related items.
Showing alternative products first helps the users find what they are looking for by analyzing the features of similar options. Once the purchase decision has been made, the cross-selling strategy with related items will help to increase the average order value, thus increasing the revenue.
Testimonial
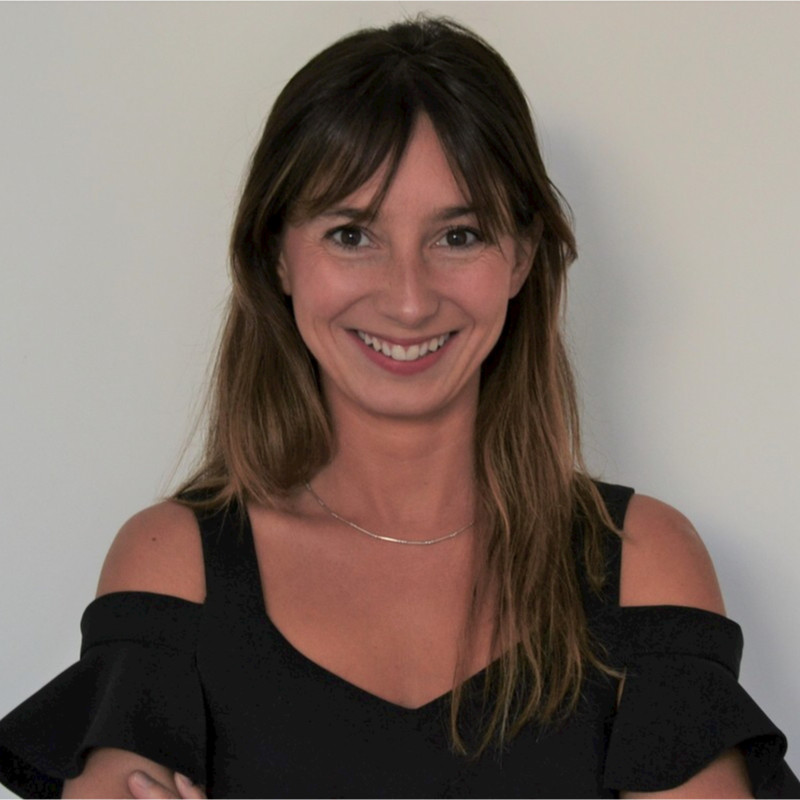
“Retail Rocket has been a significant player in helping us to personalize our sites and optimize the shopping process by providing recommendations according to our users’ preferences. They have helped us improve our conversion, orders, and shopping and browsing experience through personalized product showcases, email marketing, Push Notification, and Pop-ups, among others. They also provide us with relevant and valuable performance reports to make decisions based on the metrics and KPIs set to measure each tool”.
Catalina Lavanchy – Ecommerce sales & Marketing Manager · Komax S.A.